The Role of GenAI in Cybersecurity - Opportunities and Risks

Introduction
GenAI is reshaping cybersecurity, enhancing defenses while also posing new threats. On one hand, GenAI holds the promise to revolutionize the way organizations approach cyber security. Through advanced machine learning techniques, it can be used to predict potential threats with high accuracy, automating the detection of anomalies that might indicate a cyberattack. This predictive capability allows for more proactive security measures, where systems can not only react to incidents but also anticipate and mitigate them before they impact the system. GenAI can also automate routine security tasks, from the analysis of vast data sets for threat identification to the generation of security policies, thereby enhancing efficiency and reducing human error.
However, alongside these benefits come significant challenges. One of the primary concerns is the integrity of data privacy. GenAI systems require enormous amounts of data to train effectively, and if this data includes sensitive or personal information, there's a risk of breaches or misuse. The ethical implications are also profound; decisions made or influenced by AI in cybersecurity could have repercussions on privacy, fairness, and accountability.
In this blog, we will explore how Generative AI reshapes cybersecurity, highlighting both its potential to bolster defenses and introduce new threats. We'll explore the opportunities for proactive threat management while addressing the ethical, privacy, and security challenges posed by AI-driven cyber risks.
Opportunities of GenAI in Cybersecurity
By harnessing the power of machine learning, GenAI can revolutionize how we approach security, offering unprecedented capabilities in areas like threat detection, automation of mundane tasks, and personalized security training. These advancements not only bolster defenses but also empower organizations to stay one step ahead of cyber adversaries.
One of the most significant advantages of GenAI is its ability to enhance proactive threat detection. By learning from vast datasets, GenAI can predict potential cyber threats before they materialize. An article from CrowdStrike notes that GenAI enables a shift from reactive to proactive security measures, allowing for the anticipation of attacks based on learned patterns. This predictive capability reduces the likelihood of successful breaches by enabling preemptive security adjustments.
GenAI is also instrumental for automating routine security tasks such as streamlining incident response, automating the generation of security policies, and even assist in the creation of detailed threat intelligence reports. This allows cybersecurity teams to focus on more complex problems, thus enhancing operational efficiency and response times.
Additionally this can also be used to enhance cyber security training for personnel. By personalizing training modules based on roles, past behaviors, and common threats, GenAI can significantly reduce human error, which remains a primary cause of security breaches.
We will explore a few real-world use cases for GenAI in the next section.
GenAI Use Cases in Cybersecurity
Intrusion Detection Systems (IDS)
Organizations must vigilantly monitor their networks for signs of unauthorized access or malicious activities to protect sensitive data and critical systems. This proactive surveillance helps in early detection of intrusions, allowing for swift response to mitigate potential damage.
Anomaly Detection: AI-powered intrusion detection systems use machine learning algorithms to establish a baseline of normal network behavior. They analyze traffic patterns, user activities, and system logs to identify what is considered "normal."
Real-Time Alerts: When the system detects unusual patterns or anomalies that deviate from the established baseline—such as a sudden spike in data transfer or access attempts from unusual locations—it generates real-time alerts for security analysts to investigate.
Adaptive Learning: These systems can continuously learn from new data, adapting to evolving threats and reducing false positives over time. This means they become more effective at distinguishing between legitimate activities and potential intrusions.
Without such monitoring, organizations leave themselves vulnerable to cyber threats that can exploit weaknesses in real-time. Recent studies, like the IBM Global Security Operations Center Study from 2023, highlight significant challenges within cybersecurity operations. For instance, 63% of threats that SOC team members manually review in a typical workday are low priority or false positives, indicating a high volume of unnecessary workload. Moreover, 49% of alerts that SOC team members are supposed to review go unchecked within a typical workday, which could delay response times to actual threats. A staggering 81% of SOC team members report that manual investigation of threats slows down their overall response times, underscoring the inefficiency of current practices.
However, the integration of Generative AI into IDS systems presents significant opportunities for improvement. According to the same study, features like Automated Investigation (87% would save a lot or some time) and AI-driven analytics / risk prioritization (86%) are seen as highly beneficial by cybersecurity professionals. These AI capabilities can streamline the detection and response process, reducing the time spent on false positives.
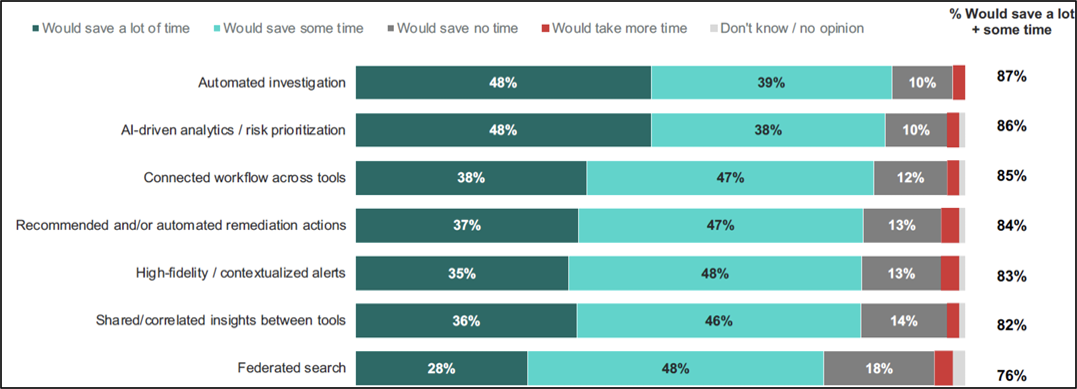
Email Phishing Detection
Phishing attacks are a common cybersecurity threat where attackers attempt to trick individuals into providing sensitive information, such as passwords or credit card numbers, by masquerading as a trustworthy entity in emails.
Unsupervised Learning: Organizations use unsupervised machine learning algorithms to analyze large volumes of email data. The algorithm identifies patterns and anomalies in email characteristics, such as sender addresses, subject lines, and content.
Feature Extraction: The model extracts features from emails, such as the presence of suspicious links, unusual language, or the frequency of certain keywords that are commonly found in phishing attempts.
Real-Time Filtering: Once trained, the model can automatically filter incoming emails, flagging or quarantining those that exhibit characteristics of phishing attempts. This helps prevent users from falling victim to these attacks.
AI and machine learning can be effectively used to enhance email security, a critical aspect of cybersecurity. By automating the detection of phishing emails, organizations can protect their users and sensitive information from potential breaches.
Fraud Detection in Banking
Banks and financial institutions face significant challenges in detecting fraudulent transactions. With the rise of online banking, the volume of transactions has increased, making it difficult for human analysts to monitor everything effectively.
Supervised Learning: Banks use supervised machine learning algorithms to analyze historical transaction data. They label past transactions as either "fraudulent" or "legitimate." The algorithm learns from this labeled data to identify patterns associated with fraud.
Real-Time Monitoring: Once trained, the model can analyze new transactions in real-time, flagging those that exhibit suspicious behavior based on the patterns it learned.
Continuous Improvement: As new types of fraud emerge, the model can be updated with new data, allowing it to adapt and improve its accuracy over time.
By automating the detection of fraudulent activities, banks can protect their customers and significantly reduce financial losses. This use of AI not only speeds up the identification of threats but also allows for a more proactive approach to security, ensuring customer trust and operational integrity.
Personalized Security Training using GenAI
Personalized security training transforms how blue and red teams prepare for real-world cyber threats by leveraging AI to simulate complex scenarios. This approach tailors learning to the individual or team's needs, fostering a deeper understanding and readiness for actual cyber engagements.
Scenario-Based Learning: Teams engage in simulations that replicate real cyber-attacks, allowing them to practice and refine their detection, response, and offensive strategies in a controlled environment.
Customized Challenges: Training is adjusted based on performance metrics, focusing on areas where teams need improvement, thus ensuring that every session is both challenging and educational.
Real-Time Feedback and Adaptation: AI provides immediate feedback during exercises, modifying the training in real-time to increase or decrease complexity as needed, promoting continual learning and adaptation.
An example of this would be the NVIDIA Morpheus framework which allows teams to train with simulated real-world threat data for realistic scenarios. Having personalized training for different roles can help enhance the efficacy of security teams when responding to real threats.
Risks Associated with GenAI from Threat Actors
The integration of GenAI into cybersecurity isn't without its issues. AI enhanced cyber attacks from threat actors are a real concern. Adversaries can use GenAI to craft sophisticated phishing emails, malware, and ransomware with speed and effectiveness. This capability dramatically lowers the barrier for threat actors, potentially leading to an increase in both the volume and complexity of cyberattacks.
Sophistication of Cyber Attacks
One of the most alarming risks associated with GenAI in the hands of threat actors is the escalation in the sophistication of cyber attacks. With GenAI, hackers can generate highly convincing phishing emails, deepfake videos, or voice clones that are nearly indistinguishable from legitimate communications.
This capability allows for more targeted spear-phishing campaigns where attackers can tailor their approach to specific individuals or organizations, significantly increasing the success rate of these attacks. The automation of malware creation is another concern; GenAI can produce polymorphic malware that changes its code to evade detection, making traditional security measures less effective.
Evasion of Detection Systems
The use of GenAI by threat actors complicates the task of cybersecurity systems designed to detect malicious activities. AI-driven attacks can learn from and adapt to security protocols, finding ways to bypass or mimic normal behavior to remain undetected.
GenAI can simulate legitimate network traffic patterns or user behaviors, making it harder for anomaly detection systems to flag these activities as threats. This adaptive camouflage could lead to prolonged undetected presence within networks, allowing attackers to establish persistent threats or conduct espionage over extended periods.
Additionally, threat actors can exploit AI to develop polymorphic malware, which dynamically alters its code signature each time it's executed on a target system. This adaptive nature of malware complicates detection efforts, as security systems must continuously update their detection methods to keep pace with these ever-changing threats.
Lower Barriers for Entry
Perhaps one of the most insidious risks is how GenAI lowers the entry barrier for cybercrime. Previously, sophisticated cyber-attacks required a certain level of expertise or access to specialized tools. Now, with GenAI, even those with limited technical knowledge can access or create complex attack mechanisms.
Platforms offering AI services or pre-trained models could inadvertently become tools for cybercrime, providing scripts or methods that automate the attack process. This democratization of cyber threats means a potential increase in the number and diversity of attackers, from lone wolves to organized crime groups, all empowered by AI technology to initiate damaging cyber operations.
Exploitation of LLMs
From an attacker's perspective, Large Language Models (LLMs) represent a goldmine for extracting sensitive information through sophisticated techniques like prompt injection and information disclosure. Prompt injection involves crafting inputs that manipulate the LLM into executing unintended commands or revealing information it shouldn't.
For example, an attacker could inject a sequence of text that appears harmless but subtly guides the LLM into accessing, processing, or even outputting confidential data. This could bypass security filters or ethical constraints built into the model, allowing attackers to gain unauthorized insights or perform actions against the system's intended protocols.
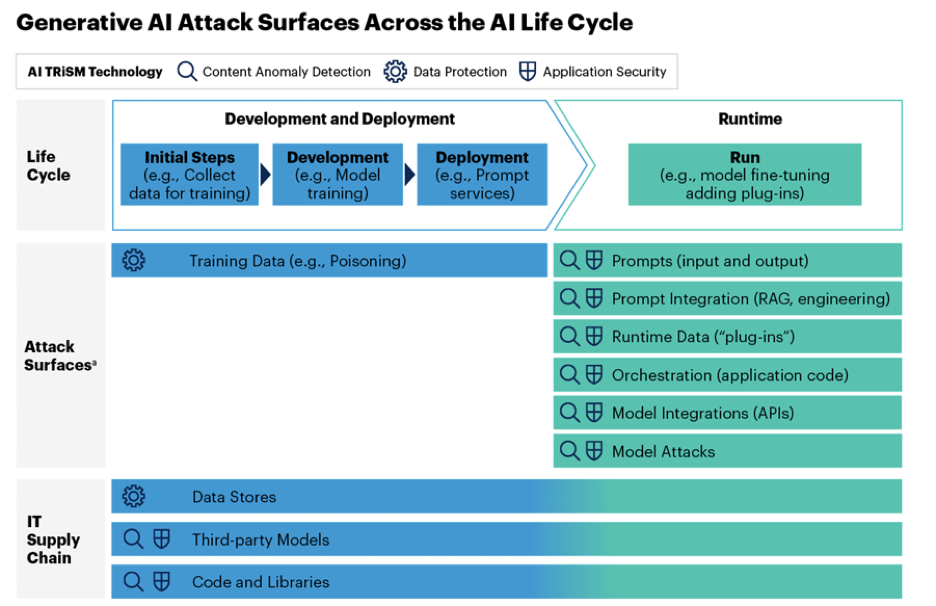
To understand the broader scope of vulnerabilities, we can look at the attack surfaces across the AI life cycle as outlined by Gartner. During the Development and Deployment phase, vulnerabilities can be introduced in several ways:
- Training Data (e.g., Poisoning): Attackers can compromise the initial training data by injecting malicious or biased information, which can skew the model's outputs over time, leading to unintended or malicious results.
- Data Stores: If the data used to train or fine-tune models is stored insecurely, attackers might access or alter this data to manipulate model behavior.
- Third-party Models: Integrating third-party models can introduce vulnerabilities if these models are not thoroughly vetted for security or if they contain backdoors or biases.
- Code and Libraries: The code used for model development and the libraries it depends on can be exploited if they contain vulnerabilities or if attackers can insert malicious code.
In the Runtime phase, the attack vectors become more direct and immediate:
- Prompts (input and output): Similar to prompt injection, attackers can exploit the model by crafting inputs that lead to outputs revealing sensitive information or executing unintended actions.
- Prompt Integration (RAG, engineering): Here, attackers might manipulate the integration of prompts with retrieval-augmented generation (RAG) to alter the context or data retrieval process, leading to misinformation or data leaks.
- Runtime Data ("plug-ins"): Plugins or additional runtime data can be tampered with to change the behavior of the model at runtime, potentially leading to unauthorized access or data manipulation.
- Orchestration (application code): The application code that orchestrates the LLM's operations can be a target for attackers to inject malicious logic or alter the flow of data.
- Model Integrations (APIs): APIs used to integrate the model with other systems can be exploited to bypass security measures or to inject malicious commands.
- Model Attacks: Direct attacks on the model itself, such as adversarial examples, can trick the model into making incorrect decisions or revealing confidential information.
Understanding these attack surfaces is crucial for organizations deploying LLMs. By recognizing where vulnerabilities can occur, security measures can be tailored to protect against these specific threats throughout the AI lifecycle, from development through to runtime.ConclusionGenAI is transforming cybersecurity by offering new ways to predict and counter threats, improving security through automation and tailored training. However, this technology also introduces risks, including sophisticated AI-driven attacks and complex privacy concerns. The challenge lies in leveraging GenAI's advantages while mitigating its potential for misuse by cybercriminals. We need to balance innovation with security, ensuring that our defenses evolve alongside the technology. Only through careful management can we safeguard our digital assets against the misuse and abuse of GenAI.
How Altimetrik Can Help
Altimetrik AI/LLM Red Teaming Service
At Altimetrik, we understand the critical importance of securing your AI systems within AWS environments. That's why we're offering our comprehensive AI/LLM Red Teaming Service designed to strengthen your AI defenses against real-world threats.
Here's how we can help:
Adversarial Testing: Let us conduct thorough testing by simulating adversarial attacks to uncover vulnerabilities in your AI models deployed on AWS.
Model Evaluation: We'll assess the robustness of your AI models, providing tailored recommendations to enhance security and performance in AWS.
Threat Landscape Analysis: Gain insights into the current threat landscape, understanding the potential risks and adversaries targeting your AWS-based AI systems.
Risk Assessment: We identify and assess risks specific to your AWS-based AI/LLM implementations, helping you minimize potential impacts.
Compliance Review: Ensure your AI systems are not only secure but also compliant with AWS-specific regulations and industry standards.
Incident Response Planning: Be prepared with our help in developing and implementing effective incident response plans for any security breaches involving AWS-based AI systems.
Security Program Development: We design and implement security programs that are customized for AI/LLM deployments within AWS.
Policy and Procedure Development: Let us create and maintain security policies and procedures that align with AWS best practices for AI systems.
Training and Awareness: Enhance your team's knowledge with our specialized training programs focused on AI security within AWS environments.
Custom Engagements: Our services can be tailored to meet the unique AWS requirements and security needs of your organization.
Detailed Reporting: Receive comprehensive reports detailing the security posture of your AWS-based AI systems, complete with risk assessments and strategic recommendations.