GenAI helps in HR Analytics: Empulse makes it better - Altimetrik
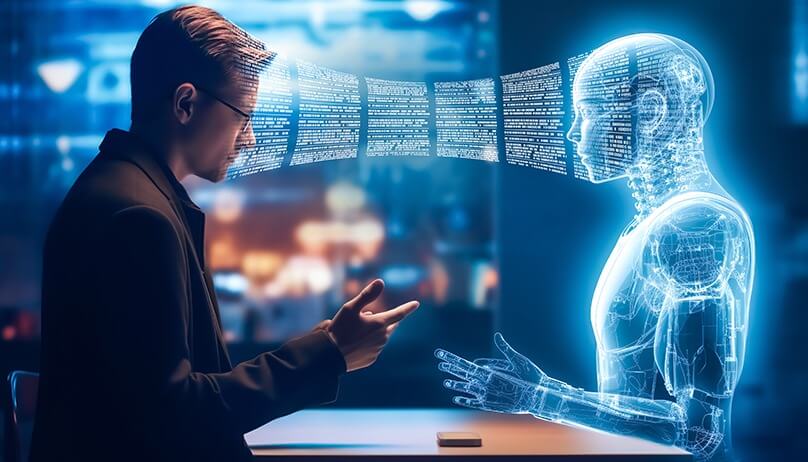
GenAI helps in HR Analytics: Empulse makes it better
The cause for HR people analytics has exponentially jumped in the recent times and GenAI extends a promising helping hand to the HR organization in processing all the big data we now have with us. Let’s look into the why, what and how of leveraging this incredible technological invention and makinga positive impact on growth as we evolve in the age of Digital Business.
Consider this – from a mere personnel management department with people typically drowning in files and papers, doing taxes and managing labor laws – the HR function has come a long way in the role we play in a business. If not directly a revenue-making centre, HR is undoubtedly the primary revenue-influencer for any business today. Why? Because highly engaged employees make a company 21% more profitable.
Employee Engagement is Crucial
The Great Resignation Wave is most likely over, but it has been an eye-opener in how we perceive a work environment, what drives people to love their jobs and stay loyal to a company. And there is no scope for a one-size-fits-all. It is an age of diversity and inclusion. The more diverse the workplace gets, the more complex employee engagement becomes. It is all in the mandate of the HR organization to understand the sentiment of their workforce and create and nurture a culture that lets people thrive. Here comes the complex challenge of garnering and understanding employee feedback.
Employee Feedback is Mission Critical
HR departments contend with a multitude of data sources, spanning third-party tools like Glassdoor, Ambitionbox, social media platforms, in-house applications, employee forums and so on. While all these sources provide insights, the unstructured nature of the data often makes it hard to derive meaningful intelligence. There is also another consideration that people in the Human Resources department are not techies, and they don’t necessarily come from a tech background. Managing all this big-data can get really difficult– a problem that GenAI aims to salvage.
But GenAI has limitations and can be tricky to use in its present state. There are reasons why we cannot entirely trust a GenAI tool and can be easily led into misinterpretations.
Here are some real-life illustrations of what happens like Hallucination, Duplication and more. We have to be cognizant of these problems before we jump into the bandwagon. This is not a mere fad that we pick up and run with.
Yet, this doesn’t mean that HR leaders should shy away from tapping into this brilliant technology which offers a smart way to deal with this fundamental requirement. To deal with theproblem and effectively leverage GenAI, we need a solution that is aware of the loopholes, paves a work-around and delivers results that are more accurate and reliable.
Empulse – a strategic framework to work around the challenges & derive accurate HR analytics
The recently launched framework by Altimetrik called Empulse – is easily deployable, meticulously crafted by experienced AI engineers and Practitioners who have invested years closely tracking the HR industry, comprehending ourchallenges, objectives, and evolutionary patterns.
For example, Altimetrik HR department was struggling with various sources of employee feedback. Especially with the new hybrid work model that we rolled out, it was important for us to get a deep dive into employee feedback to manage employee engagement better. We decided to take help from Empulse which baselined a massive amount of data from various structured and unstructured sources from Altimetrik and helped the company gain insights to frame initiatives and policies that is helping us with engagement and retention. It has taken data from our internal sources, applications, social platforms and helped us understand our employees better.
How is GenAI used in HR Analytics?
Empulse is in a good place to help HR departments useGenAI effectively, derive actionable insights into the organizational employee sentiment and positively impact retention and attrition. It can elevate our decision-making process and prioritize employee-centricity.
Technically, this framework harnesses the potency of Large Language Models (LLMs) like GPT in tandem with an intelligent pre- and post-processing engine. Empulse’s language engine transforms unstructured data into structured insights, encompassing sentiments, topics, intent, and emotions. It goes a layer deeper into the data to get more contextual and accurate analysis of the data collected from a myriad of structured and unstructured sources that makes for a smart work-around to the present loopholes in using GenAI for HR Analytics.
Harnessing the Power of HR Analytics
As leaders, we recognize the paramount importance of analytics in shaping the future of our organizations. The HR organization in a company will become even more strategic and critical in the coming years as talent is the greatest asset. Having a strong data analytics capability is a powerful tool in our arsenal that will enable us to help the business stay sharp and ahead of the curve.
As hiring continues to remain a challenge globally, retention and employee engagement are important goals that we cannot afford to run short on in the fast-changing digital world. The secret lies in the optimal synthesis of technology and the human factor striking a harmonious balance.
I eagerly anticipate the future where the synergy between GenAI and human ingenuity illuminates a brighter tomorrow. How about you?