How to set up and run Llama3 on Windows - Altimetrik
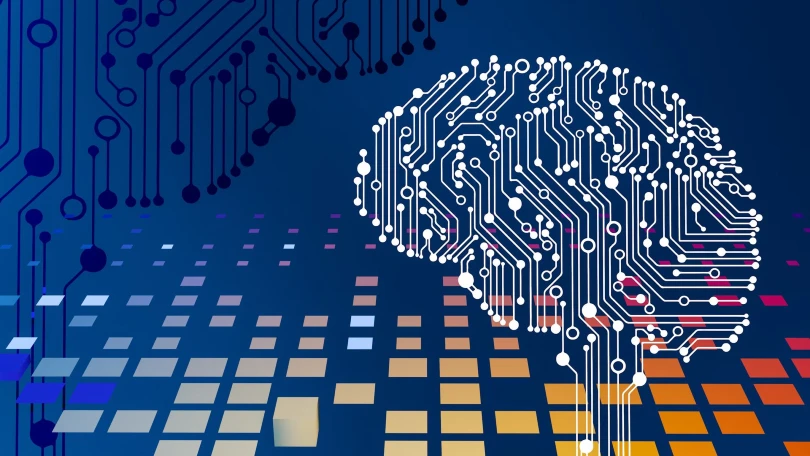
A step-by-step guide to running this revolutionary AI model on Windows!
As a fellow AI enthusiast, I’ve been experimenting with various models and frameworks for months, including Fabric from Daniel Miessler. This open-source framework is designed to augment human capabilities using AI, providing a modular approach to solving specific problems. Worth checking out here!
In addition to Fabric, I’ve also been utilizing Ollama to run LLMs locally and the Open Web UI for a ChatGPT-like web front-end. This has allowed me to tap into the power of AI and create innovative applications.
What is Llama3 and how does it compare to its predecessor?
Recently, I stumbled upon Llama3. Developed by Meta, this cutting-edge language model boasts state-of-the-art performance and a context window of 8,000 tokens – double that of its predecessor, Llama2! The Llama3 family of models includes both pre-trained and instruction-tuned generative text models in 8 and 70B sizes.
The Llama3 instruction-tuned models are specifically designed for dialogue use cases and outperform many open-source chat models on industry benchmarks. What’s more, the model has been carefully optimized for helpfulness and safety – a crucial consideration when working with AI.
Are you ready to unleash the full potential of Large Language Models (LLMs) and experience the future of artificial intelligence? If so, then join me on this exciting journey as we explore how to set up and run Llama3 on Windows!
How do I set up Llama3 on Windows? Follow these steps:
– Download Llama3: Get the latest version of Llama3 from here.
– Install Hugging Face Transformers: You’ll need this library to run Llama3. Install it using pip: pip install transformers.
– Set up your environment: Make sure you have Python 3.8 or higher installed, along with the required dependencies (e.g., nltk, spaCy).
– Run Llama3: Use the following command to load and run Llama3: python -m transformers.LLama3.
That’s it! With these simple steps, you’ll be ready to unleash the power of Llama3 on your Windows machine.
What is Open WebUI?
Open WebUI is an extensible, feature-rich, and user-friendly self-hosted web interface that operates entirely offline. It supports various Large Language Model (LLM) runners, including Ollama and OpenAI-compatible APIs.
In this blog, I’ll be going over how to run Ollama with the Open-WebUI to have a ChatGPT-like experience without having to rely solely on the command line or terminal.
Below are the steps to install and use the Open-WebUI with llama3 local LLM
1. Install Ollama
For Windows
- Download the installer here
- Right-click on the downloaded OllamaSetup.exe file and select “Run as administrator”

1. The screenshot above displays the download page for Ollama.
For Linux WSL:
NOTE: For this method, make sure to have Ubuntu WSL installed, we can easily do this by visiting the Microsoft store and downloading the latest version of Ubuntu WSL.
You must enable virtualization and Windows Subsystem for Linux in Windows Features.

2. The screenshot above displays the option to enable Windows features.
2. Install Open-WebUI or LM Studio
Check out the Open WebUI documentation here
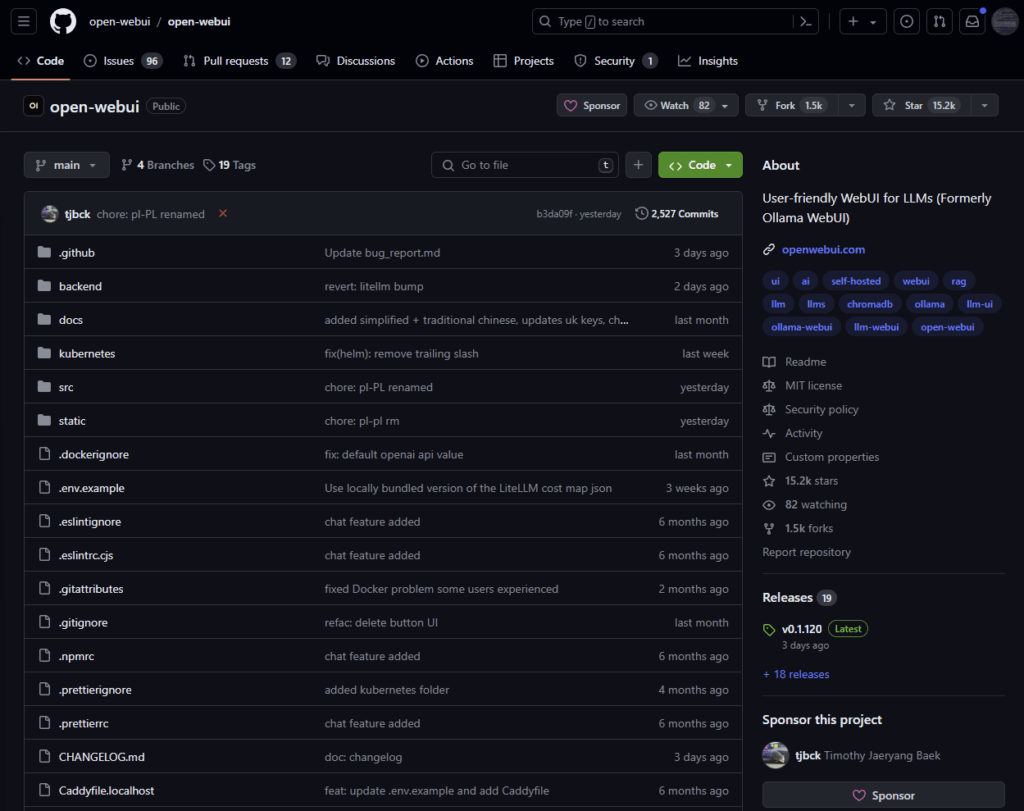
3. The screenshot above displays the GitHub page for Open-WebUI.
Check out the LM Studio documentation and download LM Studio from here

4. The screenshot above displays the download page for LM Studio.
# Run the following command in PowerShell or Ubuntu WSL:
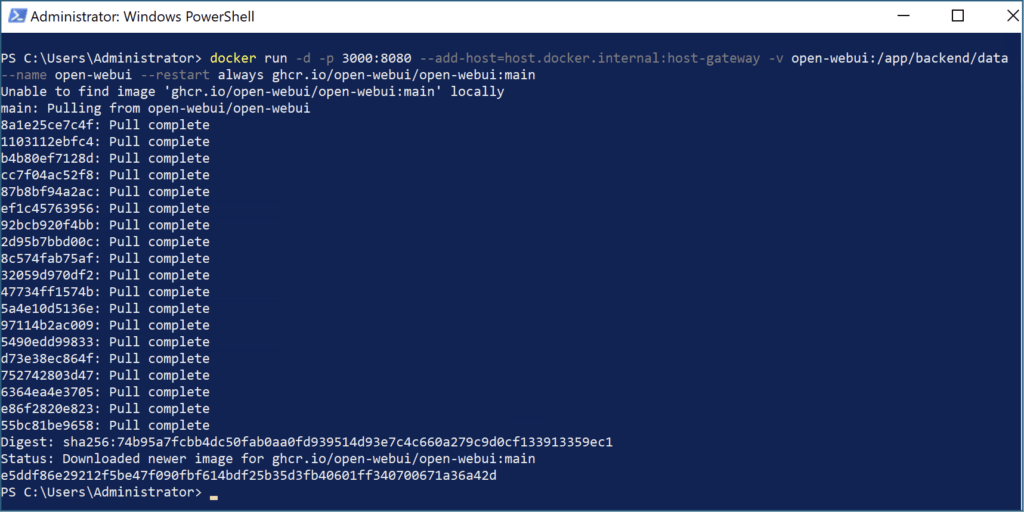
5. The screenshot above exhibits the docker run command in PowerShell.
Install Docker Desktop by following the link here
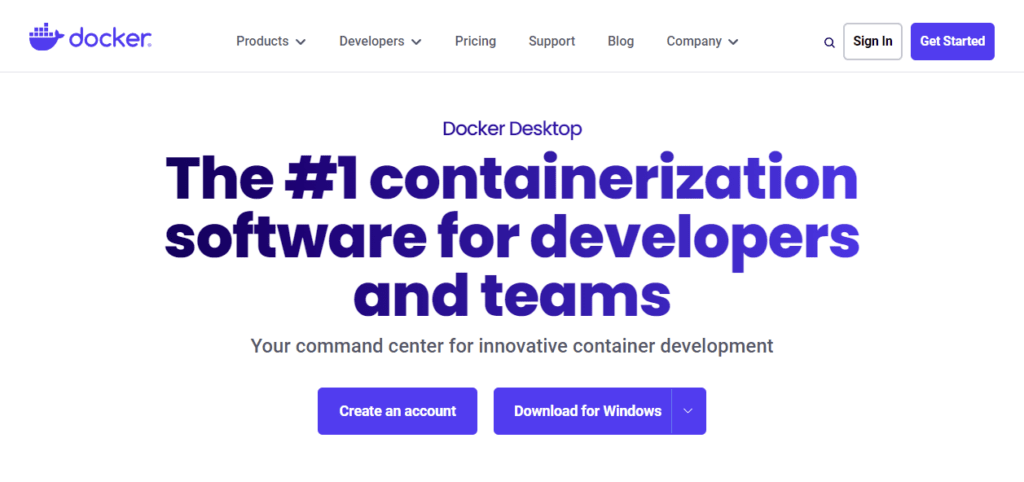
6. The screenshot above displays the download page for Docker.
Note- For Ubuntu WSL:
You must also enable WSL Integration into Docker Desktop to be able to run Open-WebUI with llama3 if you are running from WSL.
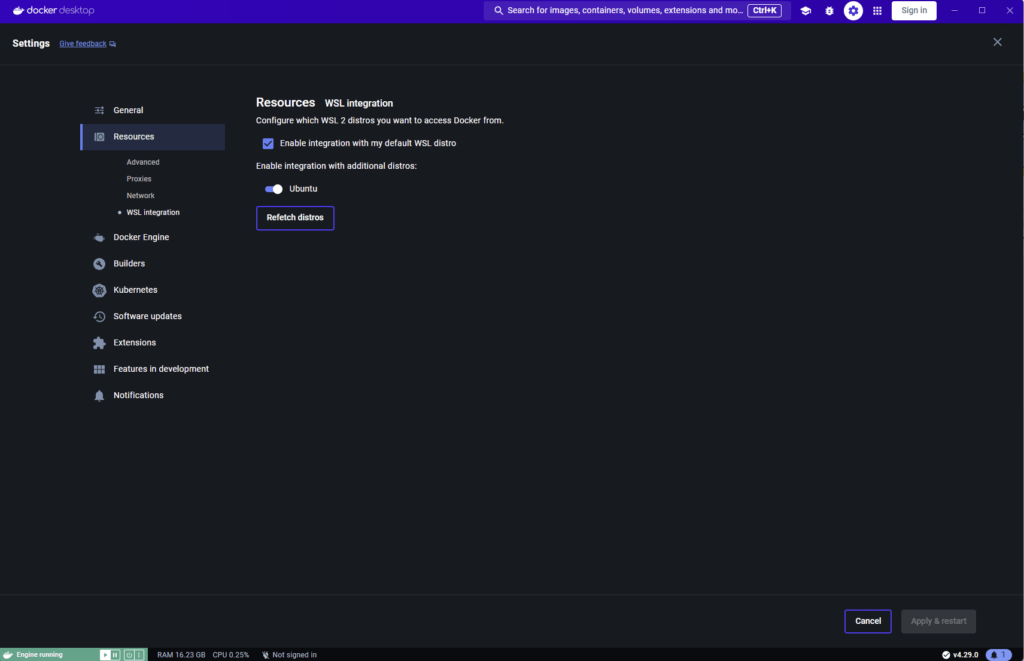
7. The screenshot above exhibits the WSL Integration into Docker Desktop.
3. Sign in to Open WebUI
Access Open WebUI at `http://localhost:3000` or `http://WSL_IP_HERE:3000`.
For the first time, you need to register by clicking “Sign up”.
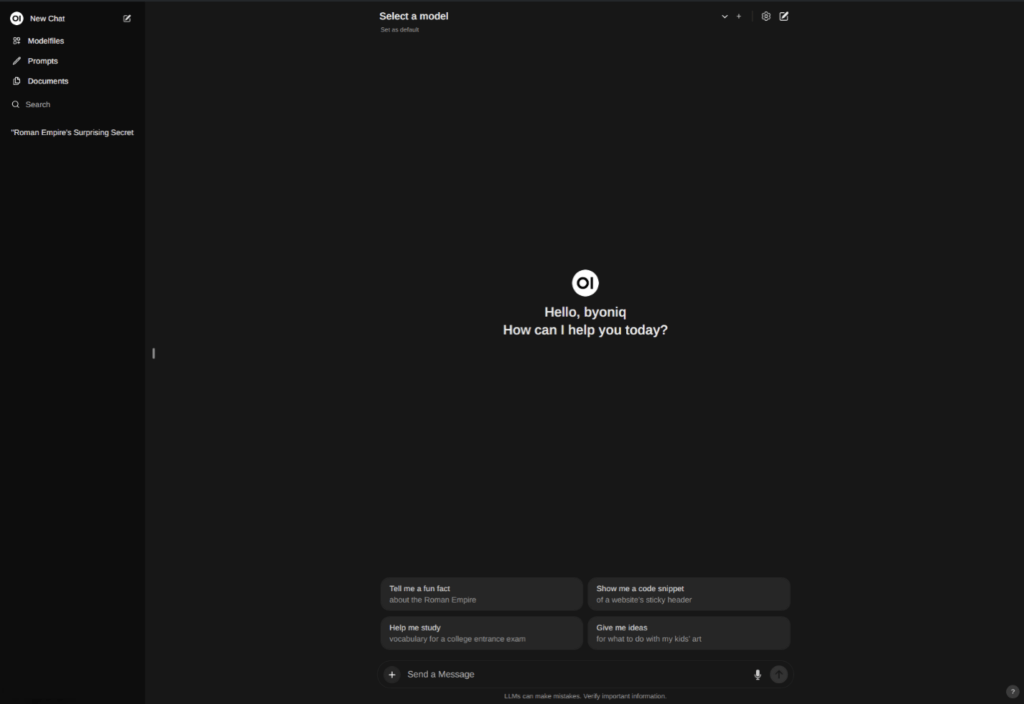
8. The screenshot above displays the home screen for Open WebUI
4. Pull a model from Ollama.com
Click the settings icon in the upper right corner of Open WebUI and enter the model tag (e.g., `llama3`).
Click the download button on the right to start downloading the model.
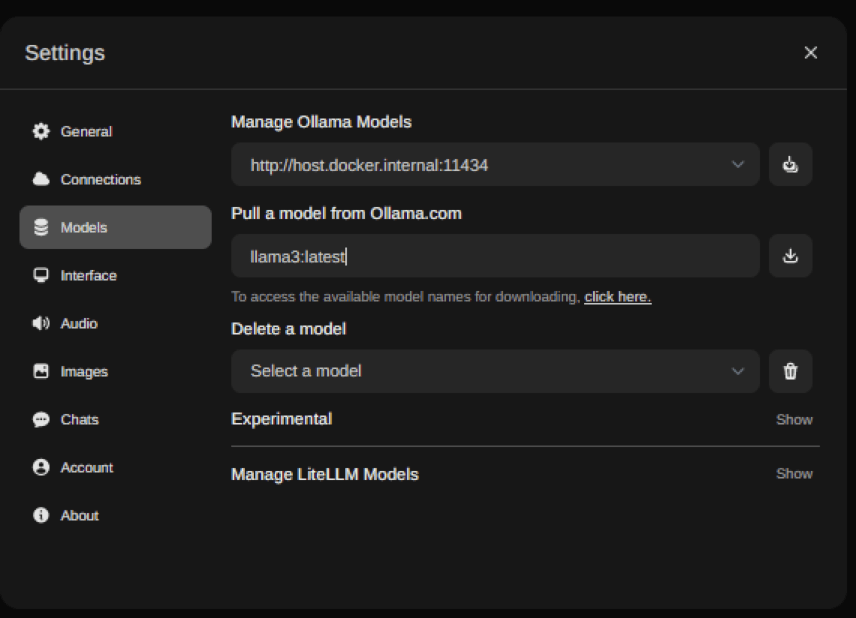
9. The screenshot above displays the settings for Open WebUI to download llama3.
5. Select a model and enjoy your AI chat

10. The screenshot above displays the downloaded llama3 module.
Here is an example of what you can do with llama3 and Open-WebUI
- You can request a Disaster Recovery Plan outline and get step-by-step instructions to formulate a cohesive and efficient plan.
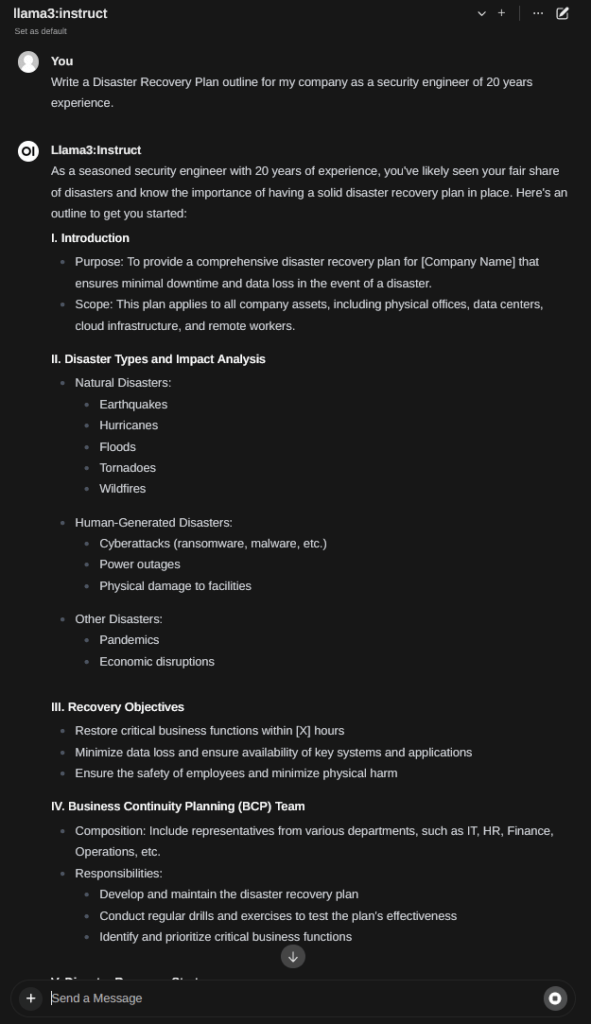
11. The screenshot above displays the response generated by llama3
2. Additionally, you can upload a document and summarize key points:
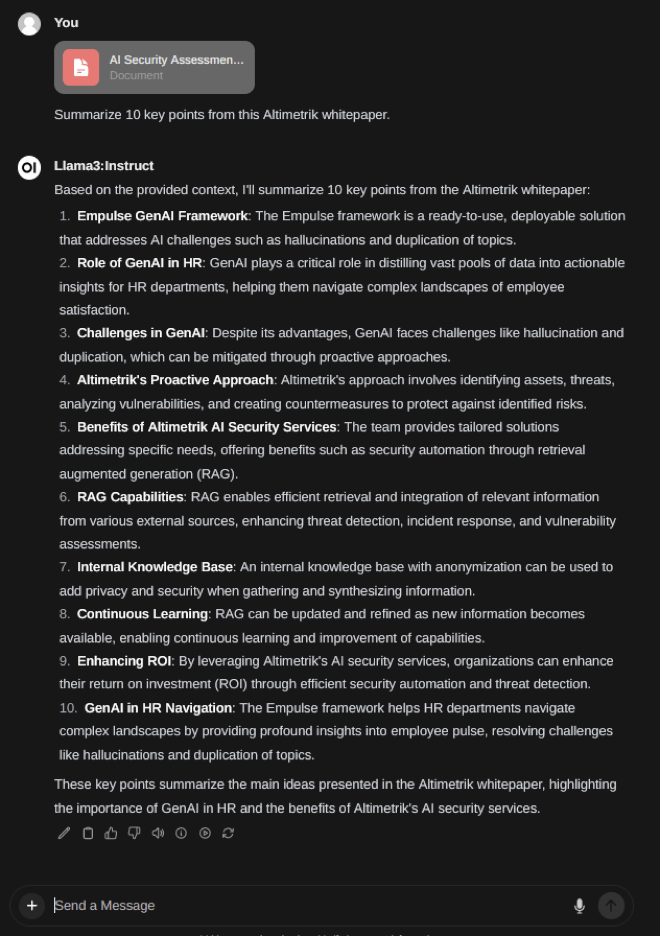
12. The screenshot above displays the llama3’s response to the uploaded document
Conclusion
Having local Large Language Models (LLMs) offers significant advantages. Local LLMs allow you to fine-tune your models using custom datasets, tailoring them to your unique objectives and tasks. This personalization ensures that your LLM aligns with your specific requirements, providing more relevant and accurate assistance.
Unlock the Power of Local Large Language Models: A Game-Changer for Data Security, Customization, and Innovation
As we’ve explored throughout this journey, having local Large Language Models (LLMs) is more than just a nicety – it’s a game-changer. By keeping your queries locally, you’re not only ensuring the security of your data but also gaining unparalleled control over your models.
Customization: The Key to Unlocking Your Unique Potential
Local LLMs also empower you to fine-tune your models using custom datasets, tailing your unique objectives and tasks.
Data Security: The Ultimate Peace of Mind
Imagine being able to ask questions and receive answers without worrying about sensitive information falling into the wrong hands. With local LLMs, that’s exactly what you get. By processing queries locally, you eliminate the risk of data privacy concerns and potential information leakage – a crucial consideration in today’s digital landscape.
I hope this guide has provided you with a comprehensive understanding of the benefits and possibilities of running local LLMs, setting you on a path to explore and harness their capabilities effectively.
As LLMs continue to advance, with models like Llama 3 pushing the boundaries of performance, the possibilities for local LLM applications are vast. From specialized research and analysis to task automation and beyond, the potential applications are limitless. With the ability to run LLMs locally, you gain control over your data and model customization, empowering you to leverage the full potential of these powerful language models while maintaining privacy and aligning with your unique needs.
Additional Resources